Predicting the Unpredictable
Last week, I took part in a Challenge Workshop on Immunopsychiatry, a branch of psychiatry that explores the connection between mental health and the immune system (the system that defends us against potentially dangerous microorganisms, such as viruses, keeping us healthy), in a wonderful location in Switzerland.
The workshop brought together the most experienced researchers worldwide to present their recent discoveries and to brainstorm all together on how to foster the state of the art of the field.
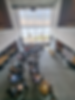
I am a psychotherapist and a researcher working at the Unit of Psychiatry and Clinical Psychobiology, Division of Neuroscience, IRCCS San Raffaele Hospital in Milano, Italy. I was invited as a junior faculty member to present my and my brilliant colleagues' research in an on-fire 15-minute talk in front of this unique audience.
You can only imagine the pressure. What psychologically helped me?
The fact that my presentation was on one of the current hottest topics: the use of machine learning to personalise treatments in psychiatry.
Talking about Artificial Intelligence (AI) is now commonplace. The whole world is engaged in creating the "perfect" AI; just note the clamour around the chatbot ChatGPT that has boosted the giants of Silicon Valley and beyond. This is also happening in mental health. But for very specific reasons associated with the limits of research in the last 20 years.
Unsolved clinical issues in psychiatry
Mental health is a severe concern in terms of public health; we have also seen it with the COVID-19 pandemic. Depression, for example, is a leading cause of disability worldwide, affecting one in twenty people, a number that is steadily increasing.
Nowadays, the diagnosis and treatment processes are mainly based on the collection of signs and symptoms and on what the clinician can observe and investigate during an interview, often when the patient is already ill. Furthermore, in most cases, patients show a mixed clinical picture, and, in many other cases, treatments are not effective in ameliorating the individual well-being, forcing several attempts before finding an effective treatment.
For instance, in depression, approximately 30% of depressed patients do not respond to popular antidepressant treatments such as selective serotonin reuptake inhibitors (SSRIs) or serotonin and norepinephrine reuptake inhibitors (SNRIs).
Why is this happening? Because the diagnosis is based on symptoms and not on biological and psychological causes. So, it can prevent the identification of the best treatment option.
For this reason, over the last 20 years, the research has moved to identify the biological and behavioural factors involved in the etiopathogenesis (that is the cause and subsequent development of a condition) of mental disorders. This includes brain structure and function, genetics, and the inflammatory profile. However, things somehow were not working.
What if it is all about heterogeneity?
Even within the same diagnosis, different people may experience different profiles of symptoms and severity.
For example, two individuals with depression may exhibit completely different symptom patterns, such as insomnia versus hypersomnia (i.e., feeling excessively tired during the day or sleeping longer than usual), or loss versus increase of appetite. Furthermore, the causes of mental health disorders are also multifactorial and can vary significantly between individuals.
While genetics play a role, also environmental factors, life experiences, trauma, and social determinants of health can contribute and interact in a very personal way to the development and course of psychiatric disorders.
So, things are not working because we are probably too unique and different among ourselves. And this is where AI comes into play, or rather a specific branch of it: machine learning.
Machine learning exploits algorithms to learn from data how to make accurate predictions and can help in harnessing the power of big data, identifying recurring or hidden patterns among billions of variables and participants. In this way, algorithms can stratify, or aggregate, patients according to their specific biological, behavioural, and psychological characteristics, building more homogeneous subgroups of patients.
With this, we can define new "diagnoses" based on these signatures, nearest to the biology and other individual features. This can help clinicians make more accurate predictions about patient outcomes and improve the early detection of mental health disorders and the development of more effective intervention strategies, tailored to individual needs: thus, a precision psychiatry.
In the near future, clinicians may be able to include further diagnostic elements, provided by supportive AI tools, to guide their therapeutic choice. Not too different from what already happens in other branches of medicine, where a physician can propose the most effective intervention based on a blood test or radiological imaging. But when we talk about the mind, diagnosis, and related solutions can be more complicated.
Ethical aspects that cannot be neglected
It is mandatory to consider the ethical issues involved when we talk about AI and machine learning, but the questions to be raised are different: from data ownership and protection to privacy, from the management of potential bias or errors in the algorithms’ training to the implications in terms of indications on treatment and diagnosis.
There is still a long way to go before being able to use these algorithms in clinical practice, but a categorical refusal of these new methodologies would be counterproductive, considering their potential benefits. New guidelines are also being defined at the European and International levels to regulate the application of this technology and its use in care processes.
Well, I was curious to see how ChatGPT would suggest concluding an article about the potential impact of machine learning in psychiatry. It replied, "In conclusion, the incorporation of machine learning in psychiatry has the potential to reshape the field, empowering clinicians with advanced tools for early detection, precise diagnosis, and tailored treatment strategies, ultimately enhancing mental healthcare outcomes."
Maybe it’s a bit scholastic but I cannot disagree with it.
